While AI offers significant benefits to healthcare, it also presents several risks that must be addressed to ensure safe and ethical implementation. Understanding these risks and developing effective mitigation strategies is crucial for maximizing AI's positive impact.
We don’t know what we don't know. AI in this stage of its development is a fairly new technology, so its long-term implications are a terra incognito for the entire industry. Proper risk management and adherence to the fundamental principles of AI TRiSM (AI Trust, Risk, and Security Management) will help businesses avoid major potential threats on this path.
While healthcare is one of the most regulated industries the world over, this is good news in the case of the Artificial Intelligence adoption. HIPAA, GDPR, the HITECH Act already have quite a few of the safeguards needed to keep patient data and other sensitive information secure.
In this series of articles on AI in healthcare, we explore key factors that make Artificial Intelligence technology so critical for the health and wellbeing of the species. I this piece, let us deep-dive into the potential negative outcomes and how to overcome them.
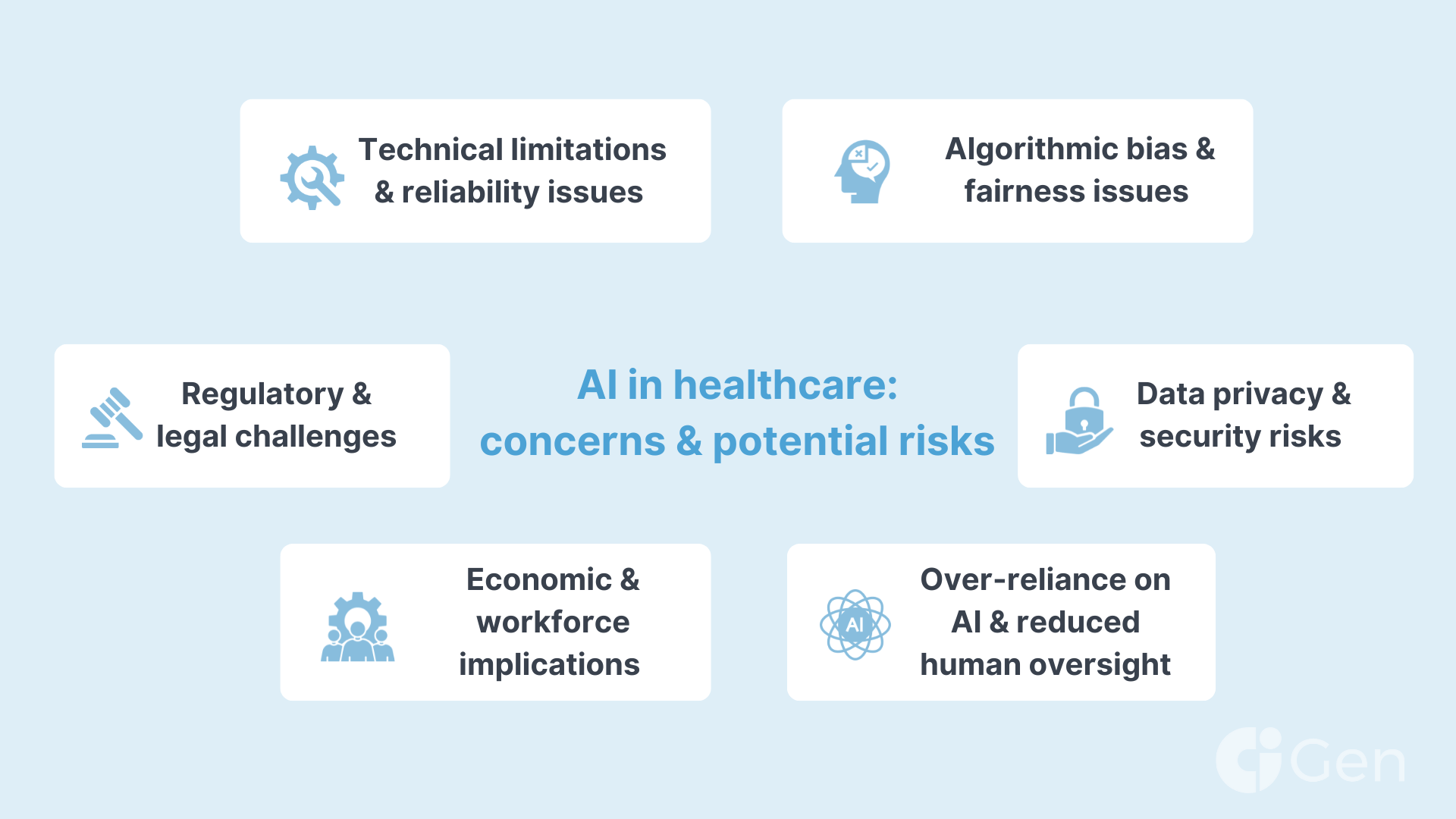
Data privacy and security risks in healthcare
Data is a valuable asset, this is why EMR (Electronic Medical Records) and EHR (Electronic Health Records) storage and processing have so many regulatory guidelines. Bigger corporations will have their own data to train models with, but when it comes to smaller companies and startups, having access to data (even anonymized) is critical.
The industry is yet to learn how to regulate, store, and exchange the data with community clouds being one of the pieces of the puzzle.
Risks:
Unauthorized access and data breaches
The extensive use of sensitive patient data increases the risk of cyberattacks and unauthorized access, potentially leading to identity theft and loss of patient confidentiality.
Inadequate data protection measures
Insufficient security protocols can result in accidental data leaks and misuse of personal health information.
Mitigation strategies:
Robust encryption and security protocols
Implementing advanced encryption methods and multi-factor authentication to protect data integrity and confidentiality is already an industry standard that is gaining even more significance now.
Compliance with regulatory standards in healthcare (HIPAA, GDPR, CCPA)
Companies need to adhere strictly to regulations such as HIPAA and GDPR, ensuring that all AI systems meet legal requirements for data protection and patient privacy.
Regular security audits and assessments
While major pharmaceutical companies have rigid internal and external security audits, this continuous audit and monitoring mindset is applicable to smaller players in HealthTech as well. Conducting ongoing evaluations of security systems helps to identify and address vulnerabilities promptly.
Data anonymization and de-identification
Due to the highly sensitive nature of health records, this PII data needs to be anonymized as early on the chain as possible, leaving the scientists and developers with pure statistical input.
Utilizing techniques to remove personally identifiable information from datasets used in AI training and analysis is expected to be widely used across the board in HealthTech.
Algorithmic bias and fairness issues
Countries with developed economies tend to have better healthcare systems with plenty of structured and unstructured health records to train models upon. The level of healthcare across different continents can be demonstrated with the life expectancy statistics as one of the factors: in LatAm countries people live 75 years on average, in Western Europe – 81, in Asia – 73 years, and in Africa – only 66 years.
States with poor health infrastructure, outdated medical equipment, and poorly digitized public healthcare ecosystems will have little info to share with AI models.
Similarly, some age groups may take their health issues more or less seriously, giving rise to another form of bias when it comes to available data.
Even culturally some nations are less prone to visit doctors as their mentality may be more stoic in essence, or more natural-remedy-inclined.
Risks:
Disparities in healthcare outcomes
Biased AI models can perpetuate and even exacerbate existing inequalities in healthcare by providing less accurate or effective services to certain populations.
Reduced trust in AI systems
Awareness of biases can lead to skepticism and reluctance among both patients and healthcare providers to adopt AI technologies.
Mitigation strategies:
Diverse and representative datasets
Companies need to ensure that training data encompasses a wide range of demographic groups and clinical scenarios to enhance the generalizability and fairness of AI models.
Bias detection and correction mechanisms
Research institutions and data scientists working in the healthcare sector should learn to implement methods to identify, quantify, and rectify biases within AI algorithms throughout the development and deployment processes.
Transparent model development
In the ideal scenario, the HealthTech industry will learn to maintain transparency in how AI models are developed and make information about data sources and methodologies available to stakeholders.
Inclusive stakeholder engagement
Another way to prevent bias is to involve diverse groups, including clinicians, patients, and ethicists, in the design and evaluation of AI systems, - to ensure that multiple perspectives are considered.
Over-reliance on AI and reduced human oversight
As humanity witnesses more instances of successful AI implementation, companies may grow to rely on Artificial Intelligence more, creating potential caveats for errors and oversights to slip into the process and outcomes.
In the medical field, human supervision is critical due to the cost of the mistake and the fact, that diagnostics and treatment are so complex and have many unique variables.
Risks:
Diagnostic and treatment errors
Excessive dependence on AI recommendations without adequate human judgment can lead to errors, especially in complex or atypical cases.
Erosion of clinical skills
Reliance on automated systems may diminish healthcare professionals' expertise and decision-making abilities over time.
Mitigation strategies:
Maintaining human-in-the-loop systems
To mitigate the over-reliance on AI in Healthcare companies will need to design AI applications to support rather than replace human clinicians, ensuring that final decisions involve human oversight and expertise.
Comprehensive training and education
Training for healthcare professionals on effectively integrating AI tools into their practice is going to be a pivotal component to maintain critical thinking and clinical judgment.
Establishing clear accountability frameworks
CAIOs of medical institutions need to focus on defining roles and responsibilities clearly, ensuring that accountability for patient outcomes remains with qualified healthcare providers.
Regulatory and legal challenges related to the use of AI in healthcare
Governmental and commercial institutions alike have their stance on AI in its early formative stages. Humanity will see more regulations and governance in terms of Artificial Intelligence, specifically in such a high-litigation industry as healthcare.
One such Pan-American initiative is a Blueprint for an AI Bill of Rights.
Below we set out the most eminent risks in legal perspective and ways to minimize negative effects.
Risks:
Lack of standardization
Inconsistent regulations and standards across regions can create confusion and hinder the widespread adoption of AI in healthcare.
Liability concerns
Determining legal responsibility for errors made by AI systems can be complex, potentially leading to litigation and financial risks.
Mitigation strategies:
Developing comprehensive regulatory frameworks
One of the key short-term missions of the industry is to develop a fair playing field for all institutions. Authorities and businesses need to collaborate with policymakers, industry leaders, and regulatory bodies to establish clear and consistent guidelines for AI in healthcare.
Ongoing policy adaptation
It is critical that policymakers update regulations continuously to keep pace with rapid technological advancements and emerging ethical considerations.
Insurance and liability coverage
Another focal point is with the insurance industry, which will need to develop appropriate insurance models and legal agreements that address liability issues related to AI usage in clinical settings.
Just like any other major pharmaceutical company, Astra Zeneca has several digital optimization initiatives in scientific and administrative departments. For example, they use Gen AI to improve the efficiency of their regulatory submissions.
Already we are seeing the benefits of generative AI from identifying novel targets to more efficient design of small and large molecules to informing clinical trial design and improving efficiency of our regulatory submissions.
Hebe Middlemiss | Director, AI Product at AstraZeneca
Technical limitations and reliability issues
As a maturing technology, AI-powered systems have many technical inconsistencies and errors to overcome.
Risks:
System failures and errors
Technical glitches, inaccuracies, or system downtimes can disrupt healthcare services and compromise patient safety.
Interoperability challenges
Difficulties in integrating AI systems with existing healthcare infrastructure can limit their effectiveness and utility.
Mitigation strategies:
Rigorous testing and validation
The first way to lower any technical risks is by conducting extensive testing under diverse conditions to ensure the reliability and accuracy of AI systems before deployment.
Robust infrastructure and support
It is vital to invest in high-quality technical infrastructure and provide ongoing maintenance and support to prevent and address technical issues promptly.
Standardized protocols for integration
As it stands right now, all major CSP like AWS, Azure by Microsoft, GCP are working to adapt their products to the AI era. Undeniably, we will see more actions to develop and adopt standards that facilitate seamless interoperability between AI applications and existing healthcare systems.
Economic and workforce implications
As AI and ML algorithms decrease the time for processing mundane administrative tasks significantly, wide adoption of this technology will inevitably lead to shifts in the workforce.
Risks:
Job displacement
Automation of certain tasks may lead to job losses or shifts in workforce requirements within the healthcare sector.
Economic disparities
High costs associated with implementing advanced AI technologies may widen the gap between well-funded and under-resourced healthcare facilities.
Mitigation strategies:
Reskilling and upskilling programs for AI engineers in healthcare
Companies will provide training opportunities for healthcare workers to adapt to new roles and responsibilities that emerge alongside AI integration.
Equitable access initiatives
Global, federal, and state healthcare institutions should aim to develop funding models and policies that support the adoption of AI technologies across diverse healthcare settings, including those in low-resource areas.
Collaborative human-AI models
Both governmental and commercial bodies in the healthcare industry can reduce the risks of negative AI impact on the workforce by emphasizing models where AI augments human capabilities, leading to enhanced job satisfaction and productivity rather than replacement.
The CAIO of General Electric's HealthCare, for example, recognizes the value of AI as the facilitator of the operational and administrative processes:
We’re driven by a mission to revolutionize healthcare interfaces by integrating voice, text, and the latest in AI visualizations. This approach isn’t just about technological novelty. It’s also about creating user-centric tools that aim to redefine how medical professionals interact with and leverage technology, improve their efficiencies, and ultimately improve patient experience and outcome.
Parminder Bhatia | CAIO at GE HealthCare
Maximizing AI's potential in healthcare through risk mitigation
While the potential of AI in healthcare is vast, from improving diagnostic accuracy to optimizing treatment plans, its implementation must be approached with care. Ensuring data privacy, reducing algorithmic bias, and maintaining transparency are critical steps in minimizing risks associated with AI.
A meticulous and well-regulated approach, combined with ongoing collaboration between technologists, healthcare professionals, and policymakers, will be essential to harness AI's full potential while safeguarding patient welfare and maintaining trust in the healthcare system.