In today’s data-driven world, the quality of your data isn’t just a technical concern—it’s a business-critical issue. Clean, reliable data forms the foundation of effective decision-making, whether you’re developing innovative AI solutions, optimizing supply chains, or driving customer engagement. Think of data like water: when it’s clean, it nourishes your operations, but when it’s polluted, it can disrupt and even damage your business outcomes.
A recent report by Research and Markets reveals that the global data quality tools market grew from USD 1.44 billion in 2023 to USD 1.61 billion in 2024. It is expected to continue growing at a CAGR of 12.04%, to reach USD 3.20 billion by 2030. This surge highlights the increasing awareness among enterprises of how data quality directly influences operational efficiency, compliance, and bottom-line results.
However, maintaining high-quality data at scale is no small feat, especially in the cloud. Challenges like duplicate records, inconsistent formats, and incomplete datasets can cripple analytics, introduce risks, and even lead to costly regulatory fines. For senior tech executives managing complex ecosystems, addressing these challenges is not just about compliance—it’s about unlocking the true potential of enterprise data.
This article will dive deep into Azure data quality tools, exploring their features, use cases, and how they integrate seamlessly into Azure’s broader ecosystem. Whether you’re building a new data architecture or optimizing an existing pipeline, Azure provides robust solutions to ensure your data is accurate, consistent, and actionable.
Why data quality matters in the cloud
In a world where businesses rely on real-time data to drive decisions, poor data quality is the silent saboteur of success. Imagine trying to navigate a bustling city with an outdated map—wrong turns and missed opportunities become inevitable. Similarly, poor data leads to flawed analytics, misguided strategies, and costly errors.
The business case for data quality
Bad data is expensive. According to Gartner, organizations estimate that poor data quality costs them an average of $12.9 million annually, impacting everything from operations to strategic initiatives. For enterprises in highly regulated industries like healthcare or finance, even minor data inconsistencies can trigger hefty fines and reputational damage.
At its core, data quality impacts:
- Operational efficiency: Duplicate or incomplete data increases the time and cost of processes like customer onboarding, supply chain management, or compliance reporting.
- Data-driven decisions: Analytics and AI systems can only be as good as the data they are built upon. Flawed inputs lead to unreliable outputs.
- Customer experience: Errors like misspelled names or incorrect addresses can frustrate customers and harm brand loyalty.
The granularity of all processes related to data processing and storage in cloud environments increase due to a variety of reasons.
Why cloud environments demand more
The shift to cloud ecosystems, such as Microsoft Azure, introduces additional challenges for data quality management:
- Volume: Cloud systems process vast amounts of structured and unstructured data, increasing the risk of inconsistencies.
- Velocity: Real-time data flows require tools that can monitor and correct issues on the fly.
- Variety: Data comes from diverse sources—IoT devices, APIs, databases—and must be harmonized to create a unified view.
Azure data quality tools are a work in progress with new features and functions being introduced on an ongoing basis as technology evolves.
Azure’s role in addressing data quality
Azure provides an ecosystem designed to tackle these challenges at scale. Tools like Azure Data Factory, Purview, and Synapse Analytics work together to:
- Detect anomalies in real time.
- Ensure consistency across diverse data streams.
- Maintain compliance with global regulations.
Maintaining high-quality data is no longer just a "nice-to-have." For senior tech executives, it’s the linchpin of a successful cloud strategy. Without robust data quality measures, organizations risk making decisions on a foundation of quicksand—a costly and avoidable mistake.
Key features of Azure data quality tools that keep giving - and keep improving
Data quality management is an ongoing process that spans profiling, cleansing, monitoring, and governance. Azure’s suite of tools is purpose-built to address these needs at every stage of the data lifecycle. Below, we explore the key features of Azure Data Quality Tools that make them indispensable for modern enterprises.
Data profiling
Azure tools enable businesses to gain deep insights into their datasets, helping uncover anomalies, inconsistencies, or gaps. This process ensures that you understand your data before deploying it for analytics or AI models.
Example: Azure Data Factory provides data profiling features that let you analyze schema, patterns, and distributions, helping you detect issues like null values or outliers early on.
While Azure Data Factory provides basic profiling within data pipelines, Microsoft Purview offers enterprise-grade profiling, metadata management, and governance features.
Data cleansing and enrichment
Cleaning data involves removing duplicates, standardizing formats, and filling gaps in incomplete datasets. Azure tools also support data enrichment by integrating external sources, ensuring richer, more accurate datasets.
Feature highlight: With Azure Data Factory, you can design workflows to clean and transform data at scale using built-in transformations or custom logic.
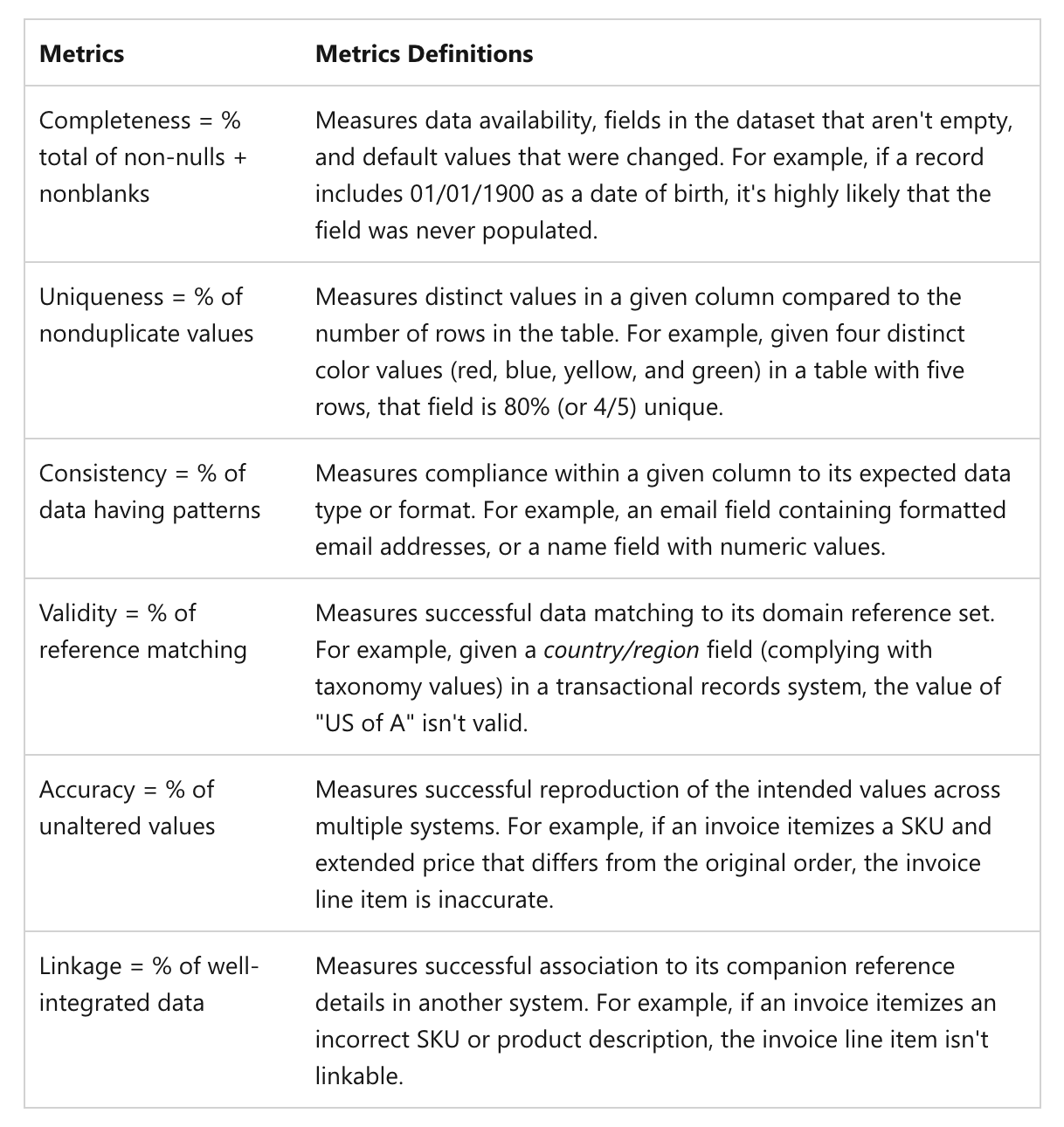
Real-time data monitoring
Azure offers the ability to monitor data quality continuously, ensuring timely detection and resolution of issues. This is particularly useful for organizations handling streaming data from IoT devices or real-time transactions.
Example: Azure Synapse Analytics integrates with Azure Monitor to flag anomalies like unexpected data patterns or latency issues.
Data deduplication and standardization
Duplicate records not only inflate storage costs but also lead to skewed analytics. Azure provides tools to identify and eliminate duplicates while ensuring data adheres to standardized formats across systems.
Example: Using Azure Machine Learning, you can create models that automate deduplication and format normalization, leveraging AI for superior accuracy.
Integration with the Azure ecosystem
Azure’s data quality tools are designed to work seamlessly within its larger ecosystem, enabling businesses to build cohesive pipelines. For instance:
- Azure Purview ensures that data governance aligns with quality standards.
- Azure Data Factory facilitates the movement and transformation of data.
- Azure Synapse Analytics enables high-performance querying on clean, consistent data.
Scalability and flexibility
Whether you’re managing small datasets or petabytes of enterprise data, Azure’s tools scale effortlessly. They also support hybrid and multi-cloud architectures, giving organizations the flexibility to adapt to evolving business needs.
Compliance and data governance
Maintaining regulatory compliance is critical, especially in industries with strict standards like GDPR, HIPAA, or CCPA. Azure tools help you document, audit, and ensure data quality meets compliance requirements.
Example: Azure Purview offers end-to-end data lineage tracking, giving you a clear view of how data flows through your systems.
Advanced analytics and AI integration
Azure integrates advanced AI/ML models to not only detect anomalies but predict potential issues in data quality. This proactive approach reduces downtime and improves overall data reliability.
Feature highlight: Azure Cognitive Services and Azure Machine Learning enable anomaly detection and intelligent tagging for enhanced quality control.
Now lets review some of the major Azure data quality tools and their key features and functions.
High-level overview of Azure data quality tools
Azure’s data quality toolkit is designed to provide comprehensive solutions for cleansing, profiling, monitoring, and governing data. Below, we’ll explore the primary tools within the Azure ecosystem, highlighting their features and how they contribute to ensuring high-quality data.
Azure Data Factory
Azure Data Factory (ADF) is a robust data integration service that enables the creation, scheduling, and orchestration of data pipelines. It plays a crucial role in data quality by facilitating:
- Data movement and transformation: Move data between on-premises and cloud environments while applying cleansing and transformation steps.
- Data profiling: Identify inconsistencies, null values, and schema mismatches before processing.
- Real-time integration: Handle streaming and batch data workflows with equal efficiency.
Use case: ADF is ideal for enterprises looking to automate data pipelines while integrating real-time data quality checks.
Azure Purview
Azure Purview is a unified data governance solution that ensures data compliance and transparency. Its data quality capabilities include:
- Data cataloging: Automatically scan, classify, and tag data sources for easier management.
- Lineage tracking: Visualize the flow of data through systems to identify bottlenecks or quality risks.
- Compliance checks: Enforce data governance policies to meet regulatory requirements like GDPR or HIPAA.
Use case: Azure Purview is essential for organizations prioritizing data governance alongside quality.
Azure Synapse Analytics
Azure Synapse Analytics combines big data and data warehousing capabilities with built-in data quality features:
- Data validation: Perform queries to validate and ensure data consistency across large datasets.
- Monitoring: Integrates with Azure Monitor to track data pipeline performance and identify anomalies.
- Integration: Seamlessly works with ADF and Purview for a cohesive data quality strategy.
Use case: Synapse is perfect for organizations managing complex analytics pipelines that require consistent, clean data.
Azure Machine Learning
Azure Machine Learning (AML) enhances data quality with AI-driven capabilities:
- Anomaly detection: Use predictive models to identify potential data quality issues before they escalate.
- Deduplication and enrichment: Leverage ML algorithms to improve data accuracy and fill gaps in incomplete records.
Use case: AML is suited for businesses that want to use predictive analytics for proactive data quality management.
Azure Monitor
Azure Monitor acts as the nerve center for tracking and maintaining data pipeline health:
- Alerting: Set thresholds for data quality metrics and receive notifications for deviations.
- Diagnostics: Analyze logs to understand and resolve quality issues in real-time.
- Integration: Works with Synapse Analytics and ADF to provide a unified view of data workflows.
Use case: Azure Monitor is essential for teams that prioritize proactive monitoring of data pipelines.
Third-party integrations
Azure also integrates with third-party data quality tools such as Informatica and Talend, allowing organizations to extend functionality where needed. These tools often bring advanced profiling, cleansing, and deduplication capabilities to complement Azure’s native offerings.
Understanding the strengths and specific use cases of each Azure tool is essential for choosing the right combination for your data quality strategy. Below is a comparative table summarizing the key features, ideal use cases, and user profiles of Azure’s primary data quality tools.
Azure data quality tools comparison
Each Azure tool offers unique strengths, and their integration provides a cohesive ecosystem for managing data quality at scale. For most enterprises:
- Start with Azure Data Factory for setting up robust pipelines with basic data quality checks.
- Use Azure Purview to ensure compliance and governance, especially in regulated industries.
- Leverage Azure Synapse Analytics for querying and validating data in large-scale analytics scenarios.
- Integrate Azure Monitor for continuous monitoring and issue resolution.
- For advanced needs, Azure Machine Learning and third-party tools can provide additional layers of precision.
Azure’s suite of data quality tools offers unparalleled flexibility, allowing enterprises to build tailored solutions that fit their unique requirements. Whether you're focused on governance with Purview, pipeline efficiency with ADF, or predictive analytics with AML, Azure has the tools to ensure your data meets the highest quality standards. Together, these tools form a robust ecosystem capable of tackling even the most complex data quality challenges.
Technical deep-dive: How Azure tools work together
Azure's data quality tools are not isolated solutions; they are designed to work seamlessly within a unified ecosystem. By integrating tools like Azure Data Factory, Purview, Synapse Analytics, and Monitor, organizations can establish an end-to-end data quality workflow that ensures accuracy, consistency, and compliance.
The workflow: End-to-end data quality lifecycle
Let’s break down how Azure tools collaborate to maintain data quality through a typical lifecycle:
Step 1: Data ingestion and transformation
Tool: Azure Data Factory
Process: ADF ingests data from multiple sources, including on-premises databases, cloud storage, and APIs. During ingestion:
- Data profiling identifies inconsistencies such as null values or outliers.
- Transformation pipelines clean and standardize data before storage or analysis.
Example workflow: Ingest customer data from a CRM system, clean invalid email formats, and transform it into a unified schema.
Step 2: Governance and metadata management
Tool: Azure Purview
Process: Once data is ingested, Azure Purview scans and catalogs the datasets, providing:
- Lineage tracking to monitor how data flows across systems.
- Classification of sensitive data for regulatory compliance.
- Metadata tagging for easier discovery and quality assessment.
Example workflow: Automatically classify customer PII data in compliance with GDPR, tagging it for restricted access.
Step 3: Validation and analytics
Tool: Azure Synapse Analytics
Process: Synapse Analytics allows users to query and validate data quality:
- Run SQL queries to identify anomalies or inconsistencies.
- Validate that transformations and cleansing steps from ADF meet business logic requirements.
- Analyze large-scale datasets with built-in analytics tools.
Example workflow: Query a data lake to ensure that no duplicate customer records exist before running BI reports.
Step 4: Monitoring and alerts
Tool: Azure Monitor
Process: Azure Monitor oversees the health of the entire pipeline:
- Sets real-time alerts for threshold violations (e.g., unexpected null values or latency spikes). Azure Monitor primarily tracks infrastructure and operational health (latency, throughput), not detailed data quality metrics. Deeper data quality monitoring requires custom logging and integration with other tools like Synapse or Power BI.
- Logs pipeline activity to diagnose quality issues as they arise.
Example workflow: Notify the operations team when a batch pipeline exceeds expected error rates, enabling quick resolution.
Step 5: Predictive and automated quality management
Tool: Azure Machine Learning
Process: AML enhances the pipeline with AI-driven insights:
- Detect anomalies using predictive models trained on historical data.
- Automate deduplication and gap-filling in datasets.
Example workflow: Use an ML model to predict missing customer demographics based on historical trends.
Visualizing the integration
A typical workflow might look like this:
- Data is ingested, profiled, and cleansed via Azure Data Factory.
- Metadata is cataloged, classified, and governed in Azure Purview.
- Cleaned data is validated and analyzed using Azure Synapse Analytics.
- Pipeline health is monitored in real-time with Azure Monitor.
- AI models in Azure Machine Learning provide predictive and automated enhancements.
When used together, Azure’s tools create a symphony of data quality processes, ensuring that your enterprise operates with trustworthy data at every step. By establishing a seamless workflow that integrates ingestion, governance, validation, and monitoring, organizations can achieve unparalleled data quality while maximizing efficiency and compliance.
Azure data quality tools: Case studies
Examining real-world applications of Azure's data quality tools provides valuable insights into their practical benefits. Below are two case studies illustrating how organizations have leveraged Azure services to enhance data quality and operational efficiency.
Parker Aerospace enhances safety with Azure Databricks
Background: Parker Aerospace, a division of Parker-Hannifin Corporation, partnered with Republic Airways to gain insights into the performance of its aerospace components during flights. The collaboration aimed to develop monitoring algorithms to improve system performance and reduce unplanned downtime.
Challenge: The company faced infrastructure limitations that hindered its ability to process vast amounts of flight data efficiently. Processing times extended up to 14 hours, delaying critical insights.
Solution: By adopting Azure Databricks, Parker Aerospace significantly reduced data processing times from 14 hours to less than two. This improvement enabled the development of a "Leak Detection Algorithm" to monitor hydraulic fluid levels, alerting maintenance teams to potential issues before they affected operations.
Outcome: The implementation of Azure Databricks allowed Parker Aerospace to process large datasets nightly, providing timely insights into aircraft performance. The integration with Power BI facilitated the creation of interactive dashboards, enhancing decision-making for both Parker Aerospace and Republic Airways.
SPAR Netherlands streamlines operations with Azure Integration Services
Background: SPAR Netherlands (SPAR NL), operating nearly 450 convenience stores, sought to streamline operations across its network by adopting a cloud-first approach to enterprise resource planning (ERP).
Challenge: The company needed to replace several legacy systems, including a pivotal automation solution not available as a cloud service. The existing infrastructure lacked transparency, making it difficult to monitor data flows and diagnose issues.
Solution: SPAR NL, in collaboration with HSO Global Services, built an event-driven, serverless integration platform using Azure Integration Services, including Azure Logic Apps and Azure Functions. This platform connected existing on-premises services with scalable cloud applications, providing end-to-end monitoring and agility to respond swiftly to changes.
Outcome: The new integration platform offered SPAR NL complete transparency into its systems, enabling quick diagnostics and insights. The architecture facilitated scalability, allowing SPAR NL to adapt to new innovations and maintain efficient operations across its diverse store formats.

These case studies demonstrate the tangible benefits of implementing Azure's data quality and integration tools. By leveraging services like Azure Databricks and Azure Integration Services, organizations can overcome infrastructure challenges, enhance data processing capabilities, and achieve greater operational efficiency. These real-world examples underscore the critical role of robust data quality solutions in driving business success.
Benefits of using Azure data quality tools
Ensuring high-quality data is not just about cleaning up errors—it’s about creating a strong foundation for better decision-making, compliance, and innovation. Azure’s data quality tools provide organizations with an end-to-end solution that enhances accuracy, consistency, and usability across enterprise data pipelines. Below, we explore the key benefits of using Azure for data quality management.
1. Improved data accuracy and consistency
Azure’s tools help eliminate duplicate, incomplete, or incorrect records, ensuring that organizations work with reliable data. With Azure Purview’s automated classification and data lineage tracking, teams can maintain a single source of truth across cloud and on-premises systems.
Example: A retail enterprise using Azure Synapse Analytics can validate product pricing and inventory levels in real-time, reducing discrepancies between online and in-store pricing.
2. Seamless integration with the Azure ecosystem
One of Azure’s strongest advantages is its native integration with other Azure services, allowing for a unified data management approach. Whether you’re using Azure Data Factory for ETL workflows, Azure Monitor for real-time issue tracking, or Azure Machine Learning for predictive quality analytics, the tools work together to provide a holistic data quality strategy.
Example: A financial services firm can integrate Azure Purview with Synapse Analytics to enforce regulatory compliance while maintaining high data integrity for analytics.
3. Scalability for big data and cloud-native workloads
As businesses grow, so does their data. Azure’s cloud-native tools are designed for scalability, ensuring that enterprises can manage data quality across vast and complex datasets without performance bottlenecks.
- Azure Synapse Analytics can process petabytes of structured and unstructured data while maintaining integrity.
- Azure Data Factory enables large-scale ETL operations across multiple data sources.
Example: A logistics company tracking global shipments can use Azure Data Factory to process millions of transactions daily while ensuring accurate and timely data updates.
4. Cost efficiency through automation
Manual data quality management is time-consuming and costly. Azure’s automation features—such as automated anomaly detection with Azure Machine Learning and real-time monitoring with Azure Monitor—significantly reduce human intervention while improving efficiency.
Example: A healthcare provider can use Azure Machine Learning to automatically detect and correct patient record inconsistencies, reducing administrative workload and improving data accuracy for medical decision-making.
5. Enhanced compliance and governance
With global data regulations becoming stricter (e.g., GDPR, HIPAA, CCPA), organizations must ensure compliance while managing vast amounts of data. Azure Purview provides end-to-end governance, auditing, and compliance tracking, making it easier to align with industry regulations.
Example: A multinational enterprise can automate data access controls and classify sensitive data using Purview, ensuring compliance across multiple jurisdictions.
6. Real-time monitoring and proactive issue resolution
Traditional data quality management often involves reactive fixes after errors occur. Azure enables real-time monitoring and alerting, allowing organizations to proactively address issues before they escalate.
- Azure Monitor provides real-time logs and alerts for data quality deviations.
- Azure Synapse Analytics enables proactive validation before data is used in decision-making.
Example: An IoT-driven manufacturing plant can use Azure Monitor to detect anomalies in sensor data, preventing faulty data from causing production delays.
7. AI and ML-Driven insights for predictive data quality
With Azure Machine Learning, organizations can go beyond basic data cleaning and implement AI-driven quality checks, predictive analytics, and automated issue resolution.
Example: A financial institution can use Azure ML anomaly detection to predict fraudulent transactions based on data inconsistencies, strengthening security measures.
8. Cross-cloud and hybrid capabilities
Many enterprises operate in a hybrid or multi-cloud environment. Azure’s flexibility ensures that data quality management is not restricted to Azure alone—it can extend across AWS, Google Cloud, and on-premises systems.
Example: A multinational corporation with both on-prem and cloud-based ERP systems can use Azure Data Factory to unify data from various environments while ensuring quality consistency.
Azure’s comprehensive data quality management ecosystem delivers accuracy, compliance, scalability, and automation, making it an ideal choice for enterprises handling vast and complex datasets. By leveraging Azure Purview, Synapse Analytics, Data Factory, Monitor, and Machine Learning, organizations can proactively manage data quality, reduce operational costs, and unlock new insights for better decision-making
Common pitfalls while using Azure data quality tools and how to avoid them
Implementing a data quality strategy with Azure tools is a powerful way to ensure consistency, accuracy, and compliance. However, organizations often encounter challenges that can undermine their data initiatives. Below are some of the most common pitfalls and practical solutions to overcome them.
1. Ignoring data quality from the start
Many organizations treat data quality as an afterthought, focusing on data storage and analytics first, only to realize later that poor data leads to poor insights.
Pitfall: Businesses ingest massive amounts of data without profiling or validating it first, leading to a "garbage in, garbage out" problem.
Solution: Establish a data quality-first approach by:
- Running data profiling in Azure Data Factory before ingestion.
- Implementing Azure Purview for automated data classification and lineage tracking.
- Using Azure Synapse Analytics to validate data before it reaches critical systems.
2. Over-reliance on manual data cleansing
Cleaning data manually is time-consuming, error-prone, and unsustainable for large datasets.
Pitfall: Teams manually deduplicate records or correct inconsistencies in Excel instead of automating the process.
Solution: Leverage Azure's automation and AI-driven tools:
- Use Azure Machine Learning’s anomaly detection to flag suspicious data patterns.
- Automate deduplication and standardization with Azure Data Factory transformation activities.
- Deploy Azure Synapse Analytics rules to enforce data validation automatically.
3. Lack of end-to-end data governance
Many companies struggle with data sprawl, where information is scattered across multiple sources, making governance and compliance difficult.
Pitfall: Data lacks visibility, making it hard to trace its origin, transformations, or usage.
Solution: Implement a governance framework using:
- Azure Purview for end-to-end lineage tracking and governance policies.
- Role-based access controls (RBAC) to restrict sensitive data access.
- Automated metadata tagging to classify and manage datasets efficiently.
4. Failing to monitor data pipelines in real-time
Without continuous monitoring, data quality issues can go unnoticed until they cause business disruptions.
Pitfall: Data inconsistencies are only detected when errors surface in analytics reports, leading to delays and incorrect business decisions.
Solution: Set up proactive monitoring with:
- Azure Monitor alerts for unexpected deviations in data pipelines.
- Automated logging with Application Insights to track anomalies.
- Power BI dashboards to visualize data health in real time.
5. Not accounting for scalability
Data quality strategies that work on a small scale may break down when data volume, velocity, and variety increase.
Pitfall: An organization designs its data pipelines for current needs without considering future data growth.
Solution: Build a scalable architecture with:
- Azure Synapse Analytics for handling massive data processing workloads.
- Serverless capabilities in Azure Data Factory to adapt to growing data ingestion rates.
- Auto-scaling in Azure Machine Learning to handle increased AI-driven data quality processes.
6. Underestimating the cost of poor data quality
Companies often fail to quantify the financial impact of bad data, leading to underinvestment in data quality solutions.
Pitfall: Decision-makers hesitate to invest in advanced data quality tools due to perceived costs, not realizing that poor data can cost millions in lost revenue.
Solution: Present a business case for data quality investments by:
- Using Azure Cost Management to measure the cost savings from automated data quality improvements.
- Demonstrating the ROI of clean data in driving better customer insights, fraud detection, and operational efficiency.
- Showing compliance-related cost avoidance (e.g., GDPR fines) by leveraging Azure Purview’s governance capabilities.
7. Assuming Azure’s built-in features are sufficient for all use cases
While Azure offers powerful native data quality tools, some scenarios may require third-party enhancements.
Pitfall: Companies assume Azure’s native tools cover all data cleansing and profiling needs, leading to gaps in functionality.
Solution: Augment Azure with third-party tools when needed:
- Informatica or Talend for advanced data profiling.
- Trifacta for interactive data preparation.
- Fivetran for automated ETL workflows alongside Azure Data Factory.
Data quality is a continuous process, not a one-time fix. Organizations that proactively implement governance, automation, monitoring, and scalability strategies will unlock the full potential of Azure’s data ecosystem. By avoiding common pitfalls and leveraging the right Azure tools, businesses can ensure their data remains accurate, reliable, and valuable—powering smarter decisions and sustained growth.

Wrapping up: Azure data quality tools for enterprise agility, efficiency, and innovation
In today’s data-driven economy, ensuring high-quality data is no longer a luxury—it’s a necessity. From improving decision-making and enhancing operational efficiency to ensuring regulatory compliance, data quality is a critical factor in an organization's success. Poor data quality can lead to misguided strategies, compliance risks, and operational inefficiencies, whereas well-governed, accurate, and reliable data unlocks business potential.
Throughout this article, we explored Azure’s data quality tools and their ability to provide end-to-end data management solutions:
- Azure Data Factory enables data ingestion, transformation, and cleansing at scale.
- Azure Purview ensures governance, compliance, and lineage tracking.
- Azure Synapse Analytics provides validation and real-time data insights.
- Azure Monitor continuously tracks pipeline performance and data health.
- Azure Machine Learning enhances data quality with AI-driven anomaly detection.
We also examined real-world case studies, common pitfalls to avoid, and the future of data quality management with AI-driven automation, real-time monitoring, and hybrid-cloud governance.
For organizations that prioritize data quality as a strategic asset, Azure provides a scalable, integrated, and future-ready ecosystem. Whether you’re handling petabytes of enterprise data, governing multi-cloud environments, or deploying AI-driven analytics, Azure’s comprehensive approach ensures that your data remains accurate, actionable, and trustworthy.
Data quality is not a one-time project but an ongoing discipline. By leveraging Azure’s powerful ecosystem, businesses can move beyond traditional data cleaning and adopt proactive, intelligent, and automated data quality strategies that fuel better business outcomes.
Is your data ready to drive your next big decision? With Azure, the answer can be a confident "yes".